Artificial Intelligence and machine learning are revolutionizing the way search engines operate. Here’s everything you need to know about how it works – and how to use it for SEO.
There has never been any ambiguity about the mission of Google Search. From the very beginning, its goal has been to “deliver the most relevant and reliable information available.” In general, this meant apply increasingly complex algorithms in an attempt to accurately parse meaning from the query term. And then Artificial Intelligence (AI) broke onto the scene. While AI tools like ChatGPT and Google’s forthcoming Bard have captured a lot of headlines in recent months, the implementation of AI into search engines has been an ongoing process for several years. In this piece, we’ll dive deeper into the use of AI in search engines, its use in predictive search and discuss the variety of ways it has and will continue to impact search engine optimization.
What is Predictive Search?
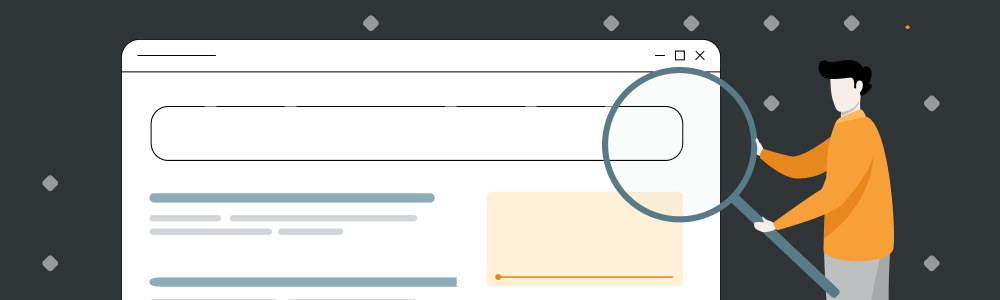
You’ve probably already noticed the use of predictive search by search engines in the form of autocomplete. That is, once you start typing a query into the search bar, you’ll often notice a list of auto-populated suggestions in a box below, as Google or your search engine of choice attempts to guess what you’re looking for. While this process provides a quite simple user experience, there’s extremely complex technology working in the background to make this possible. Essentially, the search engine analyzes every letter of your query as it’s typed and accounts for a variety of factors, including previous search behavior, geolocation, and other user attributes, as well as trending searches across users, to suggest queries while you’re typing.
AI and Searchers
The information AI uses for end users can be broken down into two larger categories: one based on user information and one based on developing an understanding of search queries. The first is fairly self-explanatory and should be familiar to anyone who has worked in search, digital marketing or a related field. But here’s a refresher, just in case: Google tracks each unique user across his or her digital activity. This includes recording demographic information, location, tracking sites visited, items viewed, and a whole host of other things. This data is then input into a complex algorithm to create a behavioral profile. The second takes a more global approach to user behaviors and factors in the behavior of many different users in returning answers to queries.
Part of this process is sometimes referred to as semantic search. This is the process search engines use to determine the intent and meaning behind queries, which in turn provides more accurate results than lexical search which uses literal matches and does not usually provide related topics. In other words, if Google has noticed users searching a specific query typically end up clicking on results from associated topics, it will begin suggesting these related sites in search results for the original query. The ultimate goal is for search engines to develop what is essentially a sixth sense about what users are seeking, so that they can recognize a vague query for “guy from band with banjo” is a search looking for information about Marcus Mumford. And the only way it can do that is by analyzing the user and global data available (like search volume and results from similar queries) to determine what that searcher likely means. Like nearly everything SEO, it’s an on-going process that will continue to be refined over time.
How Does AI Effect SEO?
Of course, it’s not just end-users who are affected by machine learning in search engines – AI also plays a large role in search engine optimization. Google uses its AI capabilities to determine how content will be ranked. It factors in things like a site’s usage of keywords and user experience scores (in other words, on-page SEO factors) to determine rankings. It’s also employed to identify black-hat SEO tactics, which is a large part of why things like keyword stuffing, cloaking and the like are now much more likely to hurt your SEO ranking than help it. But one of the biggest areas in which AI has changed search engine optimization is through voice search. With the rise in popularity of virtual assistants like Alexa and Siri, an increasingly larger percentage of queries are done via voice search. These programs use natural language processing (NLP) to recognize voices in real-time, parse their meaning and deliver relevant results. Because the goal is accuracy, this places an even stronger emphasis on content relevancy and quality than there already is. And for an SEO professional, this opens an entire world of possibilities.
Using Predictive Search for SEO
As an SEO professional, you can use predictive search to your advantage. Analyzing autocomplete suggestions can help you determine which topics people are searching for, how their searches are being phrased and uncover related concepts you may have overlooked. There are a number of helpful tools you can use to help with this, including Answer the Public and Google Trends. By entering a term or phrase, these tools provide you with a wealth of helpful information about who is searching, where they’re located, interest over time, related queries and related topics.
Predictive Search as a Keyword Research Tool
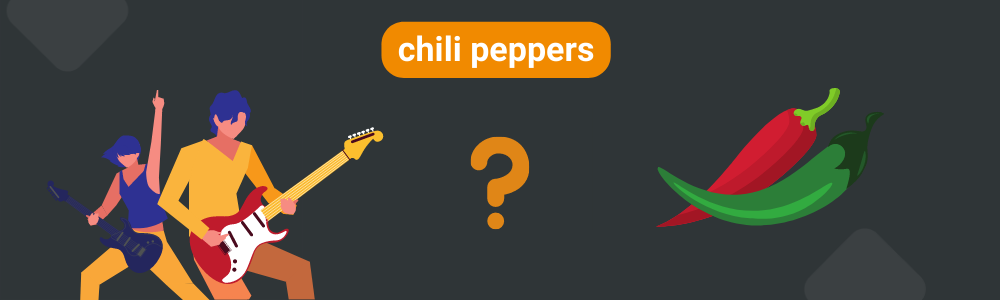
The first and most obvious benefit of predictive search for SEO is keyword discovery. While it has already shown an ability to improve the relevancy of search results, one area where it struggles is with high-level keywords. Also referred to “head keywords,” they do not often include enough information for an accurate set of returns. For example, imagine a search for “chili peppers.” Is the searcher looking for information about jalapeños? Or is this search meant to find out about the rock band The Red Hot Chili Peppers? Or maybe they’re just searching for an image of a hot pepper? It’s not only hard for humans to glean intent from that – it’s also difficult for predictive text. While search engines will have more information at its disposal about a user’s dietary habits and music preferences, there’s no way to be certain of actual intent with current technology.
Now consider a search for two keywords: “television” and “best television.” At first glance, these seem similar. But from a user intent standpoint, they’re drastically different. Someone searching for “television” is probably in the information-gathering stage of the buyer’s journey. A search for “best television,” on the other hand, is much more likely coming from someone who is in the transactional stage ready to buy. With these two examples in mind, the focus on satisfying user intent with your content cannot be understated. And this coincides with an increased emphasis on long-tail keywords – which have been gaining in SEO importance for some time anyway.
Analyzing predictive search data can help you identify new long-tail keywords to target, which in turn allows you to optimize your existing content to secure higher ranking and more clicks, as well as develop new content to target users you may otherwise have missed out on. It can also help you determine when to post new content, to ensure you’re going after keywords during peak search times. For example, focusing on a phrase like “New Year’s Party Supplies” in July is going to be far less fruitful than in December.
Predictive Search for Reputation Management
Certain businesses have bad reputations. If enough people are searching for something like “Why is XYZ company always late?” or “XYZ company product broken,” it’s going to start showing up in predictive searches. That means someone who searches for simply “XYZ company” will see all these negative autocomplete suggestions as they’re typing. In addition to driving away potential customers, this can also negatively affect your E-E-A-T, which in turn, leads to lower rankings on search engine results pages.
Key Takeaways
The goal of any search engine has always been to provide users with the results they’re looking for. AI and its sister concepts machine learning and predictive search help them hit that goal with ever-increasing accuracy. And they’re also changing the way all types of digital marketing and SEO are performed. For the immediate future, it seems likely that traditional SEO practices like backlinking, content marketing and maintaining clean site structure will remain important. However, is also appears that SEO content will continue to grow in importance for determining search results. And those sites that dive down to a more granular level and use highly specific long-tail keywords are going to find their pages at the top more and more often. Even better for those working in the SEO field, predictive search will make it easier to spot trending topics faster than ever, increasing the speed and agility with which they operate.
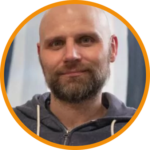
Author
Specializing in SEO, SaaS and advertising, Brian Frederick has been writing professionally for more than 15 years. A regular contributor at Search Engine Journal, his work has also appeared in the New York Times, Yahoo and the ESPN Network. He counts numerous Fortune 500 brands, including John Deere, Staples and Sprint (now part of T-Mobile) among his clients.